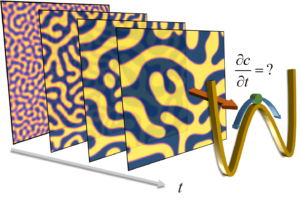
Previously we showed the possibility of learning the physics of pattern formation from images. The method enables us to learn transient and nonequilibrium thermodynamic properties in a top-down and data-driven way. However the uncertainty of the inferred constitutive laws such as free energy, diffusivity, and reaction kinetics was unknown. In a recent follow-up paper, lead author and D3BATT team member Hongbo Zhao explored the inverse problem under different conditions such as limited temporal and spatial resolution, and used Bayesian inference to quantify the uncertainty. This work paves the way for applications in the inversion of noisy experimental images of pattern-forming systems.
Read more: https://doi.org/10.1016/j.jcp.2021.110279